Oguz Tanrikulu, Director of Quantitative Research; Andrew Uhl, Senior Quantitative Analyst; and
Nastja Bethke, Senior Quantitative Researcher
April 3, 2023
Since its release in November 2022, ChatGPT has created a lot of headlines and received a lot of attention in a wide variety of professional fields. The “large language model” (LLM) developed by OpenAI has been trained on a massive dataset of text and has learned to generate human-like responses to various types of queries and prompts.
Many users have been astonished by the sophistication of its responses to fairly complex questions, leading some to proclaim the end of certain types of professions that can now be replaced by this AI.
Here we are interested in a much narrower question, namely whether ChatGPT, or algorithms like it, could be useful in systematic trading. Natural language processing (NLP) – a field that encompasses neural network-based models such as ChatGPT as well as other models, has long been used in some form or another in helping to build signals that can be used for trading stocks, futures and other financial markets. One of the simplest NLP techniques might arguably the “bag of words” approach where text is scanned for keywords that are linked – typically by the user – to a positive or negative score.
With its seemingly human-like language abilities, ChatGPT might at first glance be a much better tool to analyze sentences, as it seems to be equipped with real “understanding”, however that may be defined. We tested ChatGPT’s responses to our request to analyze 2 sentences taking from the financial news.
The first request submitted used a headline pulled from Bloomberg on 3/28/23.
1) Analyze the following sentence about gold price and provide a numerical sentiment between -100 and 100. Negative sentiment should have a negative numerical value and positive sentiment should have a positive numerical value: “Gold Slides as Hotter US Inflation Keeps Hawkish Fed on Track”.
Other than a deterministic algorithm, ChatGPT has some randomness embedded into it (at its core it autocompletes the next word in each sentence by sampling from a distribution of the most likely word to come next). This means that the same input will not lead to the same output necessarily. A couple of sample responses are shown below.
- The sentence expresses a negative sentiment towards gold price, as it suggests that the price of gold is decreasing due to the hawkish stance of the US Federal Reserve in response to rising inflation. Therefore, the numerical sentiment could be around -70.
- The given sentence expresses a negative sentiment towards the gold price. The use of the word “Slides” suggests a decline or drop in the price of gold. The sentence further indicates that the reason for this decline is the “Hotter US Inflation” which is causing the “Hawkish Fed” to stay on track. This implies that the Fed may continue to pursue policies that could further hurt the gold prices. Overall, the sentiment can be considered moderately negative. On a scale of -100 to 100, the numerical sentiment for this sentence could be around -60.
Prompting ChatGPT to respond to the request 20 times overall, we arrived at the following distribution of sentiment scores for the given sentence. The mean sentiment score was -70.75 and the standard deviation was 5.91. This seems pretty consistent and satisfactory. Note though that the input sentence is quite simple, with the key word being “slide” indicating the trajectory the price is taking. A bag of words approach should have been able to pick this up as well.
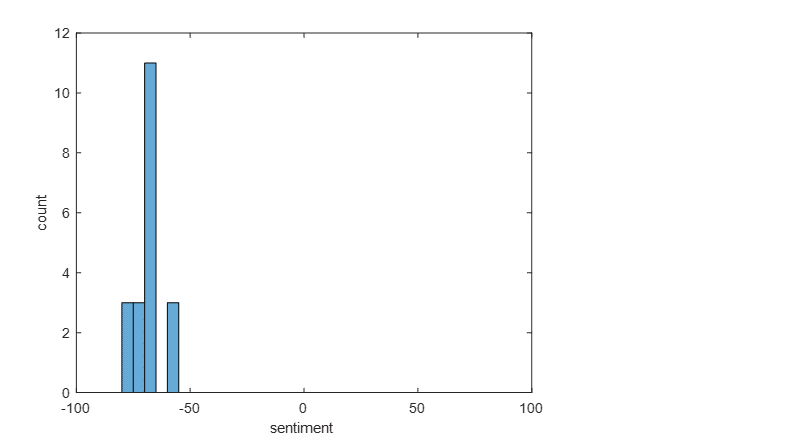
Using a somewhat more complex example, we find a different result. The second request submitted used a headline pulled from Natural Gas Intelligence on 3/24/23.
2) Analyze the following sentence about natural gas price and provide a numerical sentiment between -100 and 100. Negative sentiment should have a negative numerical value and positive sentiment should have a positive numerical value: “Natural gas futures flipped positive early Thursday after a government inventory report proved bullish relative to recent years. However, it ultimately was not enough to ease festering supply/demand imbalance concerns.”
Sample responses are again shown below.
- The sentence contains both positive and negative sentiments. The positive sentiment is that natural gas futures turned positive early on Thursday due to a bullish inventory report. However, the negative sentiment is that this positive turn was not enough to alleviate the persistent concerns over supply/demand imbalances. Overall, the sentence seems to be more negative than positive, given the persistent concerns over supply/demand imbalances. Therefore, the sentiment rating for this sentence would be around -60.
- The sentence contains a mixed sentiment where the first part expresses a positive sentiment about the natural gas futures turning positive due to a bullish inventory report. However, the second part of the sentence indicates that the positive sentiment was short-lived and the supply/demand imbalance concerns continue to persist. Overall, the sentiment can be rated slightly positive but with a note of caution due to the unresolved supply/demand concerns. Numerical sentiment rating: 40.
We find a much wider distribution of sentiment scores than before, see plot below, with a mean and standard deviation of -28.50 and 34.50, respectively. The standard deviation in this instance is larger than the mean of the distribution, making it difficult to draw any overall conclusion.
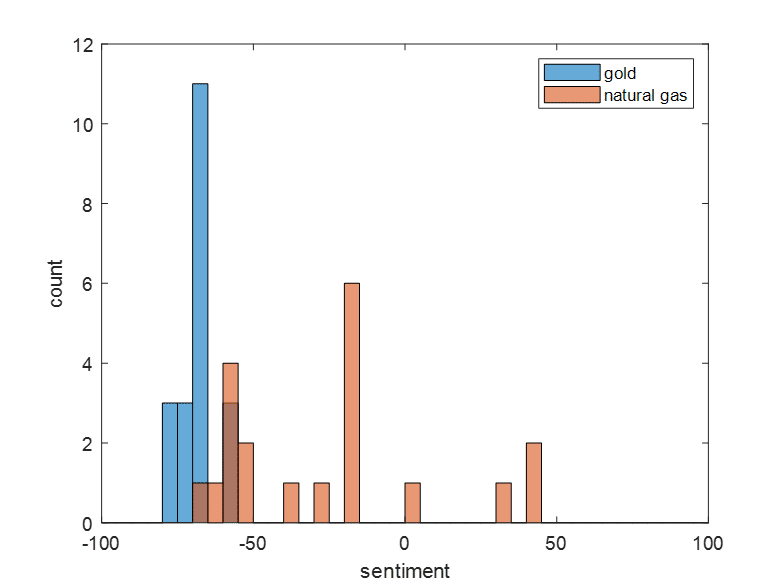
At the core of systematic trading strategies lies a design imperative of stability and robustness. The same input should give the same output, regardless of when the algorithm is run or how often, and small deviations in input should lead to small deviations in output.
Beyond this, systematic trading strategies should make transparent decisions – one should be able to rationalize output given the input. This is sometimes a bit more tricky with modern machine learning algorithms which can be highly nonlinear, but nevertheless transparency is the goal.
While ChatGPT’s capabilities are alluring, the wide range in outputs for the same input, once non-trivial text inputs are used, makes it unsuitable in its current form (and “straight out of the box”) for systematic trading.
This critique can be seen as somewhat unfair, as most humans would probably give different answers to the same question depending on when they are asked, IF the question is sufficiently vague. But then again humans do not make systematic trading decisions.